November 2018
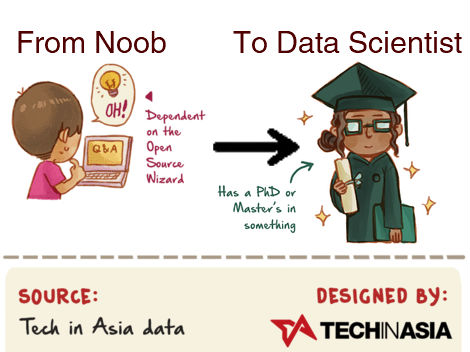
But first a quick shoutout to the image source, Tech in Asia and their 11 Types of Programmers Infographic. I'm a noob new thing evangelist who aspires to become an open source data wizard.

Quick refresher if you don't feel like revisiting my previous post, I had recommended:
- DataCamp because it is taught by famous statisticians (including my hero, Dr. Hadley Wickham) and it's free for universities IMO shows that it is committed to education over profits.
- FlatIron's free Data Science intro course because well it's free and I'm a hands on kind of person. (Did you know that you get a free month when you sign up for GitHub as a student?) But it now ranks really low on preferred resources with new information.
- SAS Programming 1: Essentials because SAS incredibly powerful and still widely used in industry, but this may not be relevant if you're settled in your current position or know you'll be using other technologies.
I Discovered the Joy of MOOCs
I still remember when MIT launched OpenCourseWare, which has since been replaced by EdX. For the longest time I didn't realize that you could audit EdX and Coursera for free.I re-discovered EdX when I heard netizens buzzing about the CS50 courses. People of all skill levels take CS50, even industry professionals. Auditing the course reminded me of a conversation I had with a classmate in undergrad. He had taken several community college courses, and was really struggling with our 2nd semester intro course. In his words, it was as if they took a year of community college and crammed it into one semester. I feel like CS50 took my first year of undergrad and put it into one course. It's the right balance of challenging and practical. I strongly recommend CS50 for beginners who are exploring whether Computer Science and / or programming is right for them, and also those of us who have been out of school for a long time and just need a refresher.
I did not discover Coursera until my coworker, a PhD, said that she wanted to use it for continuing education but just didn't have the time. I was surprised because I didn't think advanced degree holders had anything to gain from MOOCs. Until recently I didn't know that there was a difference between Coursea and other online learning platforms like Lynda (free for TAMU students), Pluralsight, Udemy, or Udacity. And while I dislike how structured Coursera can be (I'm a full time employee and part time student! There aren't enough hours in the day left.) Coursera is much more reputable because the content is developed in partnership with other universities.
- Probability - The Science of Uncertainty and Data by MITx is among one of the most highly recommended statistics courses
- Calculus Applied! by HarvardX is commonly recommended for those who need a calculus refresher. Arguably Calculus is not as important for data science, but it's hard to gain depth in statistics without a solid foundation in calculus.
- Machine Learning by Andrew Ng, former head of Google Brain, is one of the most well-known data science courses. Rumors say that it is becomming outdated and is getting replaced by fast.ai.
- courses.fast.ai by Rachel Thomas and Jeremy Howard, who dream of democratizing AI education
- Stanford Engineering Everywhere lost funding over a decade ago, but I'm using it to make sure my fundamentals are sound my preparation for my next M.S.
This semester I was supplementing my education with Querying Data with Transact-SQL because I wanted to earn a Microsoft 70-761 Certification, and also because I secretly like collecting certificates. Regardless, it's nice to have extra resources.
A Treasure Trove of Open Source Textbooks
My cohort knows that books are my favorite! I high key sitting through a video and watch everything at 2x speed. Books are just faster, and easier to reference.- An Introduction to Statistical Learning with Applications in R by Gareth James, Daniela Witten, Trevor Hastie and Robert Tibshirani is very well known and commonly used as a textbook. This is commonly referred to as an introductory text for...
- Elements of Statistical Learning by Trevor Hastie, Robert Tibshirani, Jerome Friedman a widely used textbook but considered more advanced.
- American Institute of Mathematics approved open textbooks cover a wide breadth of topics and has been previously recommended by my favorite mathematics professor.
- MIT Press Open Access There are too many good resources here to list individually, but very well known are Ian Goodfellow's Deep Learning as well as Sutton and Barto's Reinforcement Learning.
- Hadley Wickham's Works are available under the Teaching section of his website.
- Allen B. Downey's Works often recommended for his book Think Bayes, all of his works are available through his website.
- Al Sweigart's Works most well known for Automate the Boring Stuff with Python, all of his works are creative commons license.
- Packt Free e-book of the day I might just be a book horder. Don't judge. Sometimes you get lucky and it's a technology you've been curious about but didn't want to purchase the book. Most of the time the book isn't as relevant though.
Evans Library is my Favorite
There are a lot of closed source textbooks that are available digitally through our library. To name a few,- Python for Data Analysis 2e by Wes McKinney, the original founder of the pandas library.
- Data Science from Scratch by Joel Grus, who is an excellent teacher and so fun to read.
- Multilinear Algebra by W.H.Greub because sometimes you just want to learn more about tensors.
The Democratization of Education is Coming: Why You Still Need an Advanced Degree
My fatal weakness is that I am spread too thin. I want to learn everything, even if it's theoretical, obscure, or bleeding edge. I regret that I never truly understood Turing Machines and I've been pestering the Houston Linux Users Group about assembly language. Even my professor told me to slow down because I do not need to aquire all the knowledge I want right this second. I'm no stranger to self-study but I know that I would be lost without guidance. Even if you dedicated all of your time, it could still take years to read through and master all of these resources I listed. But there would be no one to answer your questions, correct your misunderstandings or mistakes.Needless to say, I based a lot of these recommendations on word of mouth. While they're all reputable and I did scope out the resources myself, I did not have the time fully audit them. At TAMU M.S. Analytics the content is expertly curated to maximize your learning for the time spent. Yes, it's rigorous and it can be time consuming but I'm confident that I will join our alumni at the top when I graduate with my ring. One quick note though, the program does not dive as deep into Computer Science as it does Business Analytics and Statistics. This is great for both beginners and experts. Beginners because the courses assume that you're entering the class with no knowledge, but also experts because the focus of the degree is on new skills. I may be breezing through the python section because of my background, but I know I will need my classmates' support next semester when it's time to dive into finance and marketing. The program may not be a good fit for you if you feel like you need a deeper Computer Science understanding than what is offered and are not willing to self study to obtain the additional knowledge. I suggest our Statistics Departments' computational statistics track or doing Computer Science with a depth in Machine Learning and Deep Learning depending on how you want to specialize. I strongly believe you need a balance of both statistics and CS, so if you already have a B.S. in computer science I personally think an advanced degree in Statistics is a better fit if data science is your goal.
Please do not go to a bootcamp without a formal education. The last meetup I attended, the buzz was that Iron Yard had closed down. It was technically old news, but I recognized the name from radio advertisements, right around the time I graduated. I remember being tempted because I didn't feel prepared for industry. All of the promises of career placement and interview training hit me at my most vulnerable.
"Also worth noting: that of those surveyed by Course Report, 60% already had bachelor's degrees. Arguably, this makes the bootcamp certification more of an addition to the college degree than a substitute for one. And this complicates any discussion of credentialing and hiring - does someone land a programming job because she or he has a college degree or because she or he has a coding bootcamp certificate?" - http://hackeducation.com/2017/07/22/bootcamp-bust
Through my own graduate admissions process, I observed that completion of a boot camp is considered more of a prerequisite than an end goal. I'm (mostly) joking here but grazing the surface of the field like that is a great way to get stranded on the "ignorance peak" of the Dunning Kruger effect.
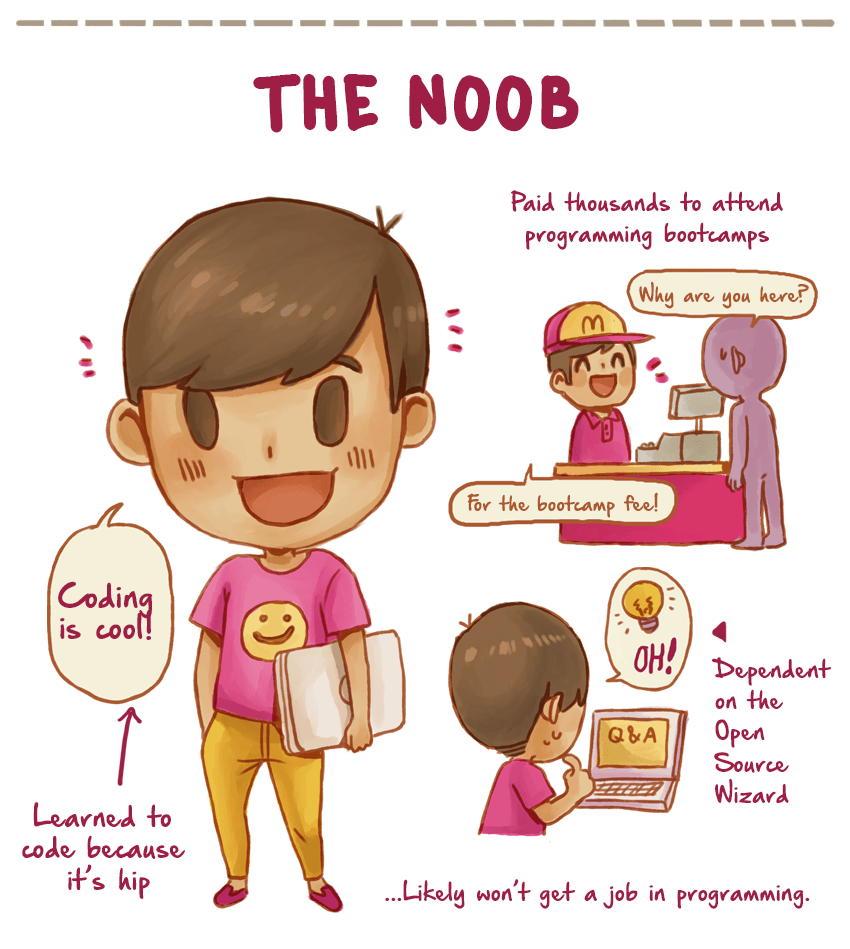

But firstly, who has time for an immersive boot camp? Not to mention the cost. The average price of these things are 15k and up. You could literally get a full M.S. for that same amount, and it's more reputable. Getting your M.S. Analytics costs a little more, but it is IMO the best value choice for a multitude of reasons. There are enough of them to whole seperate post.

You hear from many famous educations and professionals that their mission is democritization: in education, data, and AI (my personal area of interest). Knowledge is priceless, and in many ways free. But you cannot replace 1 on 1 guidance from your industry expert professors, and you cannot put a price on the value of the Aggie network. I'm hoping to welcome you into the 2021 cohort and maybe meet you at a future TAMU M.S. Analytics Info Session.
---
Jennifer Cai
Jennifer is a Masters student in the College of Science's Analytics program.